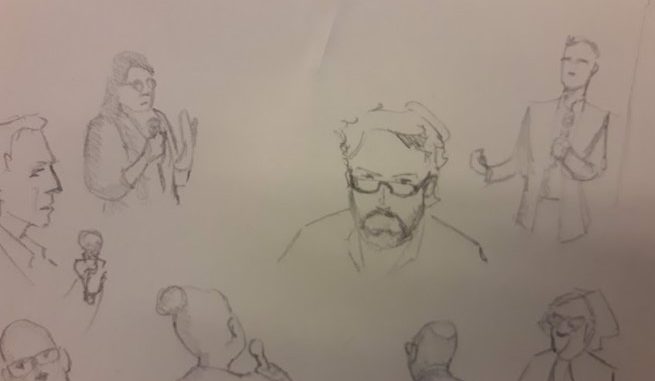
Le 8ème European University-Business Forum 2019 s’est tenu à Bruxelles les 24 et 25 Octobre 2019 (https://europa.eu/newsroom/events/8th-european-university-business-forum-ubforum_fr ). Les débats concernaient les relations entre universités et industries, en particulier vis à vis de l’intelligence artificielle.
Voici -en Anglais- la présentation du workshop ainsi que le compte rendu qui en a été rédigé.
WORKSHOP 4.2
Artificial intelligence and automisation – opportunities and threats.
This workshop will discuss the opportunities and threats for higher education, triggered by the developments and use of artificial intelligence and automisation.
Moderator: Fabrizia Benini , Head of Unit F4 – Digital Economy and Skills, DG CNECT, European Commission
Speakers:
Colin de la Higuera , Nantes University, France
Markus Lippus , Co-founder, MindTitan, Estonia
Ann Nowé, Vrije University of Brussels, Belgium
Ilkka Tuomi , Meaning Processing Ltd., Finland
1.1.1 Workshop 2: Artificial Intelligence and automation – opportunities and threats
This workshop was moderated by Fabrizia Benini , Head of Unit F4 – Digital Economy and Skills, DG CNECT (European Commission). The presentations in this workshop at the opportunities and threats for universities and businesses, caused by the developments of in the fields of automation, AI and big data.
The first speaker was Colin de la Higuera of the University of Nantes (France). Mr de la Higuera gave an overview of a report published on 22 July 2019 upon request of UNESCO on the implications of AI on education, and which skills teachers should acquire.[1] He highlighted:
- Data is inconsistent. The quality and functioning of AI is dependent on the quality of the data it gets fed. Data is never perfect, always contains contradictions and has a certain degree of uncertainty. This is important to keep in mind when relying on information provided by AI.
- Randomness. “The world is becoming more and more deterministic through better controlling randomness”. In the world of data, however, nothing is every 100% certain, and it is important to understand the impact of randomness in AI. He gave the examples of studying for an examination: is it not because you study for an exam, that you will necessarily pass the exam.
- Coding and computational thinking. It is impossible to teach AI without teaching coding. To be able to code means, to be skilled at problem-solving, using and testing data and ideas.
- (Computer-assisted) critical thinking). In a world where the borders between true and false have become increasingly blurred, it has become increasingly important to understand how computers work in order to distinguish truth from fiction. For example, it becomes very hard to distinguish ‘man-made’ from ‘computer-made’ products.
- Post AI humanism. AI has had an impact on our understanding of the world, and four notions in particular:
- Intelligence: AI is modifying our understanding of intelligence: for example, it is no longer necessarily the ability to process large amounts of data, but rather the ability to think critically and other ‘soft’ skills.
- Experience: ‘real’ experiences are disappearing and changing, becoming more digital or artificial in nature.
- Creativity: this is no longer a human feature alone. Certain AI systems can also create art or write fully-fledged stories.
- Truth: is becoming increasingly blurred, as AI has the potential of taking on previously felt to be only ‘human-like’ abilities such as bluffing, lying and deceiving.
The second speaker was Markus Lippus, Co-founder of MindTitan[2] (Estonia). His presentation focused on the work of MindTitan, as well as the data skill sets that are needed from an entrepreneurial perspective. MindTitan is a development agency working with a number of industries across a range of countries, providing them with AI and machine learning solutions.
He argued that the job of a data scientist is not much more than problem-solving with a certain set of tools. A data scientist needs to be able to formulate solutions for data problems into a language that will make the software understand how to solve them. He then extrapolated this to what scientists and researchers in general. He argued that what makes a good scientist is not the specific IT or subject-specific skills, but rather the transversal skills such as critical thinking, creativity and problem-solving.
Next, Prof Ann Nowé of the AI Lab at the Vrije Universiteit Brussel (Belgium) gave an overview of the different kinds of projects her research centre was working on. She started her presentation by saying that, back in 1965, when AI first entered the scientific discourse, AI related to modelling knowledge and research processes so that machines could stimulate, emulate or replicate tasks which were typically associated with human activities.
She then went on to explain the difference between the ‘symbolic’ and ‘hybrid’ approaches to AI. In the symbolic approach, the idea is that one tries to understand the exact logic and ‘syntax’ of an expert’s language of reasoning, and then tries to translate this into a language for the AI system to copy. In the hybrid AI approach, by contrast, one also tries to look at the sub-symbolic level. This is important if we are to build transparent, explainable and responsible AI systems which follow certain ethical and moral codes.
Ultimately, Prof Nowé argued, the decision to automate certain processes will be based on humans’ decision on whether or not this is ethical or not, and which kinds of AI errors we are ok to allow. She finished her intervention by arguing that, at present, our education systems teach History, Biology, Chemistry, Geography, etc to teach us about the world. This should, however, also include more on the digital world.
The last speaker was Ikka Tuomi, Chief Scientist and Founder of Meaning Processing Ltd (Finland). Mr Tuomi presented on the future of skills and jobs for data-driven computing, i.e. trying to link AI to learning theories.
He started his presentation by proposing ‘data-driven adaptive computing’ as an alternative term for AI, as it comes down to the ability to (quickly) process large amounts, data and the ability to derive statistical regularities from it. He then started his comparison of human learning and AI by first looking at the nature of human activity. According to Mr Tuomi, all human activity can be reduced to three levels (see Figure xxx):
- Cultural level: this is the top-level with value-driven, institution-driven and ‘common practice-driven’ levels of human activity, whereby humans engage in activities asking themselves ‘why am I doing this activity?’. For example, ‘I say “thank you” when someone gives me something, because it is common practice to do this in this or that particular country, context or situation’.
- Cognitive level: this is the middle-level, word-driven, concept-driven and sign-driven levels of human activity, whereby the question humans would ask themselves is ‘what am I doing?’. For example, ‘when I say “thank you” this means I am talking’.
- Behavioural level: this is the bottom-level, routine-driven, reflex-driven or habit-driven levels of human activity, whereby the question humans would ask themselves is ‘how am I doing this activity?’. For example, ‘in order for me to produce the words “thank you”, I need to activate my vocal chords and move my lips, tongue, etc in this or that specific way’.
Figure 3.1 Three levels of human activity (Tuomi 2019)
Mr Tuomi explained that if cultural and cognitive level activities are more social in nature, cognitive and behavioural level activities are more physiological. AI then, he argued, is able to operate at the bottom and middle levels of human activity, i.e. activities at the ‘reflex’ or ‘behavioural’ level which take less than one second of thinking; and activities at a more ‘cognitive’ and ‘symbol’ processing level. At the top level, he said, there is no AI. These are all types of human activity which relate to innovation, knowledge creation and social learning (see Figure xxx).
Figure 3.2 The ‘human potential’ of AI (Tuomi 2019)
Next, Mr Tuomi expanded on the complexity of competence, comprising four overlapping dimensions (see Figure xxx). He argued that epistemic and non-epistemic knowledge are influenced by the cultural and material context in which they are embedded. Traditionally, schools focused a lot on epistemic knowledge, i.e.: the transfer of knowledge, skills and experience. The cultural context (values and motives, norms and rules, social resources and power structures of society) as well as the material context (developments in digital technologies, and AI in particular) have had made schools focus more on developing students’ non-epistemic knowledge and meta-cognition, involving their behavioural repertoire or wider set of ‘soft skills’.
Figure 3.3 Data-driven computing in the complexity of competence (Tuomi 2019)
He then underlined that AI automation is data-biased. A lot of this data comes from the behaviours and texts written by ‘intelligent humans’, from sensors, transactions, etc. Since there is so much data, this means that data-driven computing is now not only possible but also necessary, because it can link global production processes and human activity in real time. This means we need to rethink education also. To this end, Mr Tuomi referred to his publication prepared for the JRC on ‘The Impact of AI on Learning, Teaching and Education’.[3] He also mentioned they were now working on an AI Handbook for Teachers’, developed in collaboration with teachers.
After the presentations the participants were able to ask questions to the presenters on different issues relating to the threats and opportunities of AI, big data and automation for the future of education and business. The key messages emerging from the discussions are:
- There is a need to upskill teachers on AI and computational thinking. At the moment, there is still a lot of resistance in the teacher community to learn about AI and computational thinking. It is important to change their mind-sets and make them understand that it is about learning about problem-solving in a slightly different way. A lot of efforts are being made in this respect in the UK, France and some Federal States in Germany.
- Learning to code and learning about AI develops a broad set of skills. By teaching how to code and better understand AI in general, people gain a broader set of analytical, problem-solving and transversal skills, which can be applied across a range of other disciplines. It is also important to make people understand that machines are not necessarily something to be afraid of. As an example, one participant said that “many machines still struggle to recognise cats”.
- The ‘danger’ of automation, and its impact on values and norms. Developments in big data and AI technology have society’s perceptions on the categorisation of ‘human’ and ‘computer’ activities. At the same time, despite the increasing creativity and autonomy of algorithms, it is important to remember that AI can be controlled, and there is no reason to fear technology.
- We need to rethink the way we learn. Finally, the workshop participants all agreed that rethinking education is more than rethinking curricula alone. It is about rethinking the way we learn, and how AI should be integrated in teaching methods.
[1] De la Higuera, C. A Report about Education, Training Teachers and Learning Artificial Intelligence: Overview of key issues. UNESCO and University of Nantes. Available at: http://cdlh7.free.fr/UNESCO/Teaching_AI-cdlh.pdf
[2] See https://www.mindtitan.com/
[3] JRC (2018). The Impact of AI on Learning, Teaching and Education: Policies for the future. Luxembourg: Publications Office of the European Union. Available at: https://ec.europa.eu/jrc/en/publication/impact-artificial-intelligence-learning-teaching-and-education